Edge AI (Artificial Intelligence at the Edge)
2 What is Edge AI?
Edge AI refers to deploying artificial intelligence algorithms directly on devices located at the “edge” of the network close to the data source rather than in centralized cloud servers. These devices can process data locally, often in real-time, without relying on constant internet connectivity.
Examples: Smartphones, drones, cameras, IoT devices, autonomous vehicles, wearable health monitors.
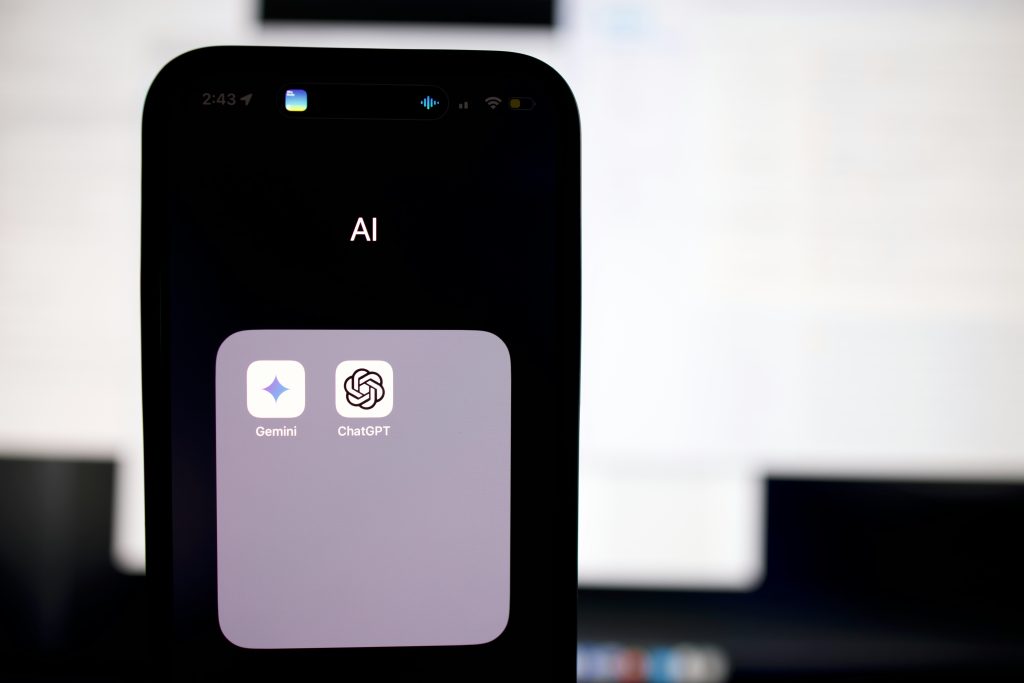
3 Core Technologies Enabling Edge AI
1 TinyML: Machine learning optimized for microcontrollers and low-power devices.
2 Edge TPUs and NPUs: Specialized hardware accelerators (e.g., Google Edge TPU, NVIDIA Jetson, Apple Neural Engine).
3 On-device inference: Running pre-trained AI models locally using optimized frameworks like:
4 TensorFlow Lite
5 ONNX Runtime
6 PyTorch Mobile
7 Core ML
4 Edge Computing Frameworks:
1 Azure IoT Edge
2 AWS Greengrass
3 OpenVINO (Intel)
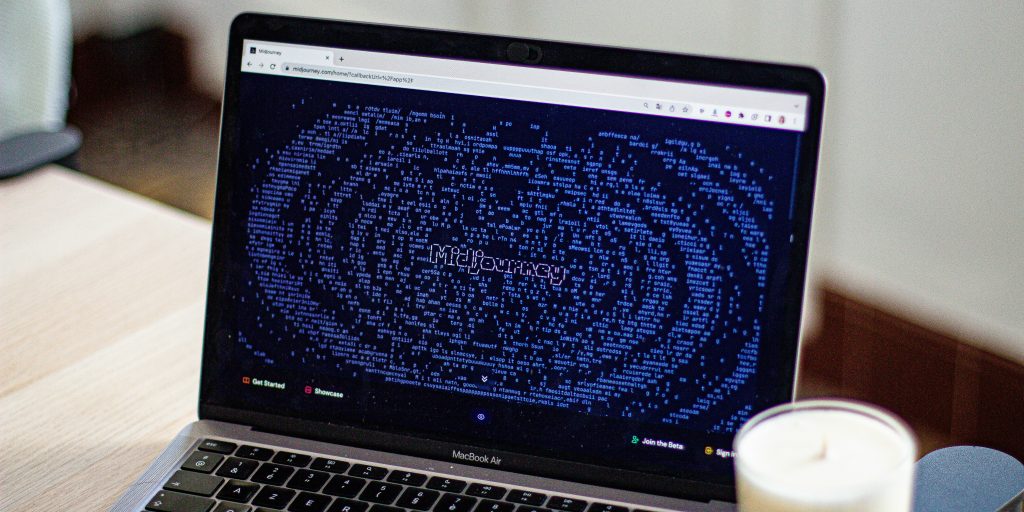
5 Challenges in Edge AI
1 Hardware Constraints: Limited memory, power, and processing power on edge devices
2 Model Compression Needs: Large AI models must be quantized/pruned/distilled
3 Device Management: Updating, monitoring, and maintaining models across many devices
4 Security Risks: Physical tampering and local data breaches
5 Standardization: Lack of unified frameworks for deployment and interoperability
6 Future Trends
1 Federated Learning: Training models across decentralized devices while preserving data privacy
2 AIoT (AI + IoT): Smart, autonomous edge devices in industry and homes
3 5G + Edge AI: Ultra-fast connectivity enabling more powerful distributed AI systems
4 Neuromorphic Computing: Brain-inspired chips offering ultra-low-power on-device intelligence
5 AutoML for Edge: Automated tools to generate edge-optimized models