Using AI to Combat Cybersecurity Threats in Real Time
2 Introduction
1 Thesis Statement: As cyberattacks grow in frequency and sophistication, artificial intelligence has emerged as a critical defense tool, offering real-time threat detection, response automation, and adaptive security strategies.
2 Context: Traditional cybersecurity methods are no longer sufficient against evolving threats like ransomware, phishing, and zero-day exploits AI offers a dynamic, proactive alternative.
3 Why Traditional Cybersecurity Falls Short
Volume and Complexity of Threats:
1 Organizations face millions of threats daily, far beyond human capacity to monitor manually.
Speed of Attack:
2 Modern cyberattacks can infiltrate systems within seconds, requiring instantaneous responses.
Evolving Tactics:
3 Threat actors constantly change techniques, making rule-based security systems obsolete without constant updates.
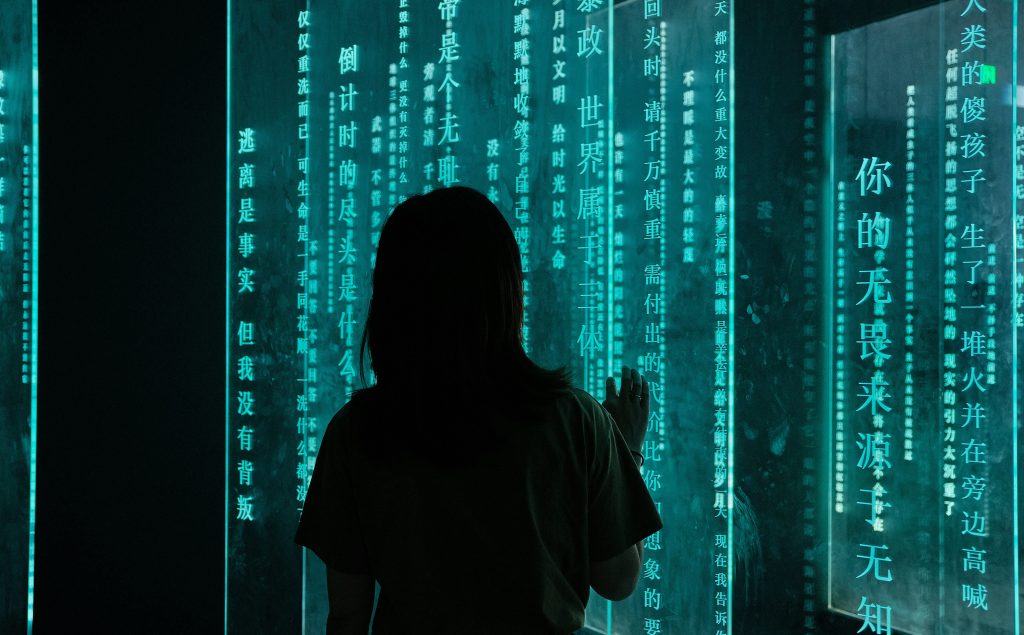
4 How AI Enhances Cybersecurity
Anomaly Detection with Machine Learning:
1 AI learns normal patterns of behaviour and flags deviations useful in identifying unknown or zero-day threats.
Behavioural Analysis:
2 AI tracks user and system behaviour to detect insider threats or compromised accounts.
Automated Threat Response:
3 AI can quarantine affected systems, block suspicious IPs, or disable compromised accounts in real time.
Threat Intelligence and Prediction:
4 Natural language processing (NLP) scans the dark web, news, and forums to anticipate upcoming threats.
Malware Detection:
5 AI can analyze code to identify malicious patterns without needing previously known signatures.
5 Real-World Applications and Tools
SIEM and SOAR Platforms:
1 Systems like IBM QRadar, Splunk, and Palo Alto Cortex XSOAR use AI to manage security data and orchestrate responses.
Endpoint Protection:
2 Tools like CrowdStrike and SentinelOne use AI to detect and respond to endpoint anomalies.
Email Filtering and Phishing Prevention:
3 AI tools scan for suspicious phrasing, spoofed addresses, and malicious links in real time.
Financial Sector:
4 AI is heavily used to detect fraud and monitor unusual transaction patterns.
6 Challenges and Limitations
False Positives and Alert Fatigue:
1 AI systems may produce too many alerts, overwhelming human analysts.
Adversarial AI:
2 Hackers are developing techniques to deceive or manipulate AI systems (e.g., through adversarial inputs).
Data Privacy:
3 Collecting behavioral and system data can raise privacy concerns, especially in regulated industries.
Bias in Detection Models:
4 Poorly trained models may overlook threats or disproportionately flag certain behaviours as malicious.
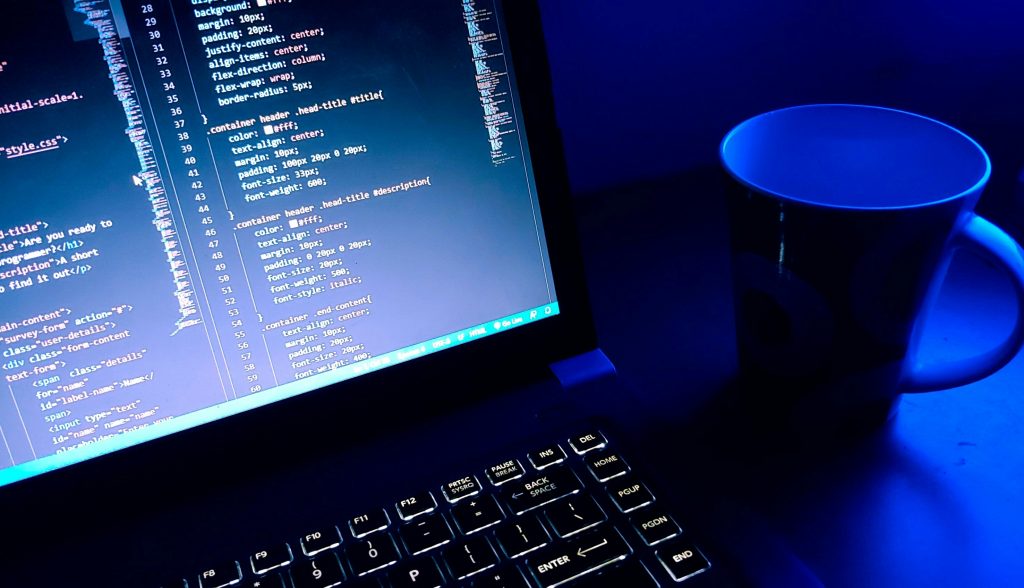
7 The Future of AI in Cybersecurity
Self-Healing Systems:
1 AI may evolve into systems that not only detect and respond but autonomously repair and adapt security frameworks.
AI vs. AI:
2 As attackers begin to use AI, defenders will need increasingly sophisticated AI to keep pace.
Human-AI Collaboration:
3 The most effective cybersecurity strategies will blend AI’s speed and scale with human judgment and oversight.
Conclusion
Final Thought: AI is not a silver bullet, but it represents the most promising evolution in the fight against cyber threats. With proper implementation and oversight, it can empower organizations to respond faster, smarter, and more effectively before the damage is done.