Cultural Bias in AI Art
2 Where Cultural Bias Comes From
AI art models (like those used in DALL·E, Midjourney, or Stable Diffusion) are trained on massive datasets of images and captions scraped from the internet. These datasets often:
1 Overrepresent Western and Eurocentric perspectives, especially in fashion, beauty, and art history.
2 Reflect stereotypes (e.g., associating certain professions or roles with specific genders or ethnicities).
3 Lack sufficient examples of non-Western art forms, traditional styles, or underrepresented cultures.
The result: AI-generated art may consistently portray certain identities in narrow, clichéd, or even offensive ways.
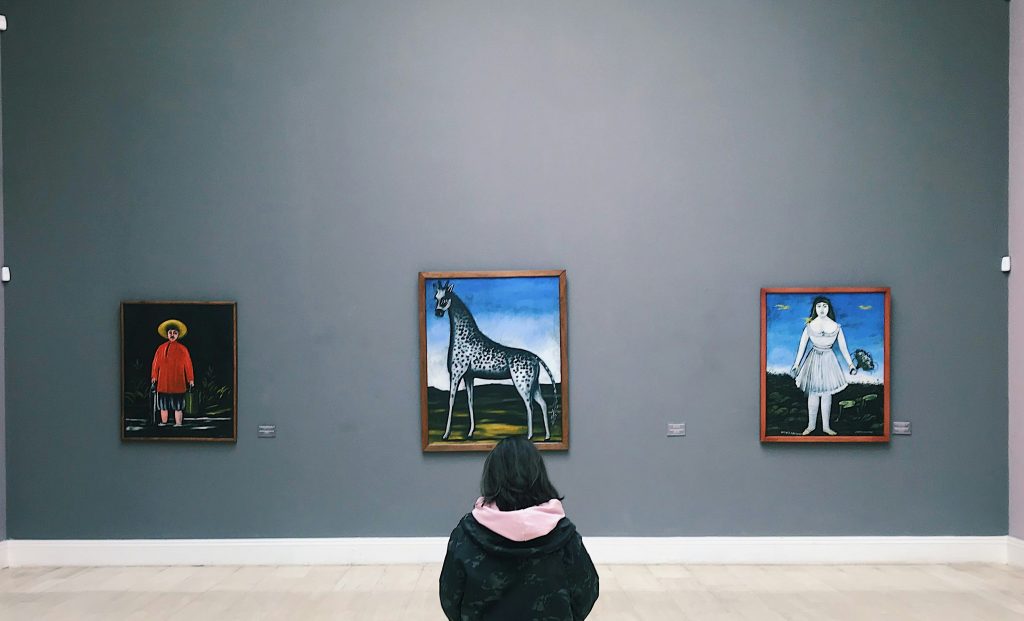
3 Examples of Bias in AI Art
1 Ethnic homogenization: Prompts like “beautiful woman” may return mostly white, Westernized faces.
2 Misrepresentation: Traditional cultural elements may be distorted or combined inaccurately (e.g., mixing elements from unrelated Asian cultures).
3 Underrepresentation: Indigenous art styles, African aesthetics, or Southeast Asian iconography are often poorly rendered or missing entirely.
4 Cultural appropriation: AI may remix sacred or culturally significant imagery into stylized art without context or respect.
4 Why It Matters
1 Cultural erasure: Lack of diversity in AI art can marginalize or invisibilize non-dominant cultures.
2 Reinforcement of stereotypes: AI outputs can amplify biases already present in society and media.
3 Ethical concerns: Using cultural symbols or styles without permission or understanding can be exploitative, especially when monetized.
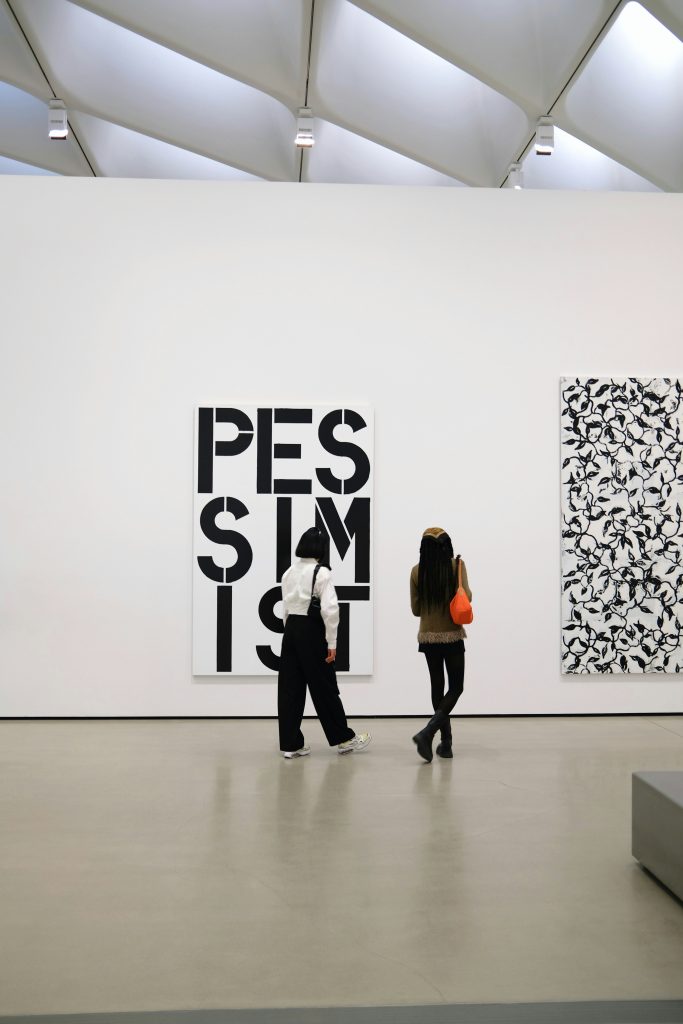
5 What’s Being Done
Some initiatives and researchers are addressing these issues by:
1 Diversifying datasets: Curating training data that better represents global cultures.
2 Bias audits: Systematically evaluating how different demographics are portrayed.
3 User control: Letting users guide style, ethnicity, and cultural elements more accurately in prompts.
4 Community involvement: Engaging artists and cultural experts in dataset creation and model development.
6 What Artists and Users Can Do
1 Be intentional with prompts and aware of what cultural elements you’re invoking.
2 Support ethical AI tools that prioritize inclusivity and transparency.
3 Credit source cultures when inspired by or referencing their styles.
4 Encourage platforms to allow flagging of problematic outputs and improve representations.
Conclusion
Cultural bias in AI art isn’t just a technical problem it’s a reflection of societal inequalities and oversights. Addressing it requires conscious effort from developers, artists, and users alike to ensure that AI art tools respect and represent the full diversity of human culture.