Bias in AI Algorithms: Can Machines Be Truly Fair?
2 Introduction
1 Thesis Statement: While AI systems are often viewed as objective, they can inherit and even amplify human biases. Achieving true fairness in AI requires critical scrutiny of data, design, and deployment practices.
2 Context: From hiring algorithms to facial recognition, biased AI outcomes have raised concerns about justice, equality, and accountability.
3 What Is Bias in AI?
Definition:
1 AI bias refers to systematic and unfair discrimination in algorithmic outcomes based on attributes like race, gender, age, or socioeconomic status.
Sources of Bias:
1 Data Bias: Historical data may reflect societal prejudices.
2 Algorithmic Bias: Choices in model design, feature selection, or optimization criteria.
3 User/Deployment Bias: How and where AI is implemented or interpreted.
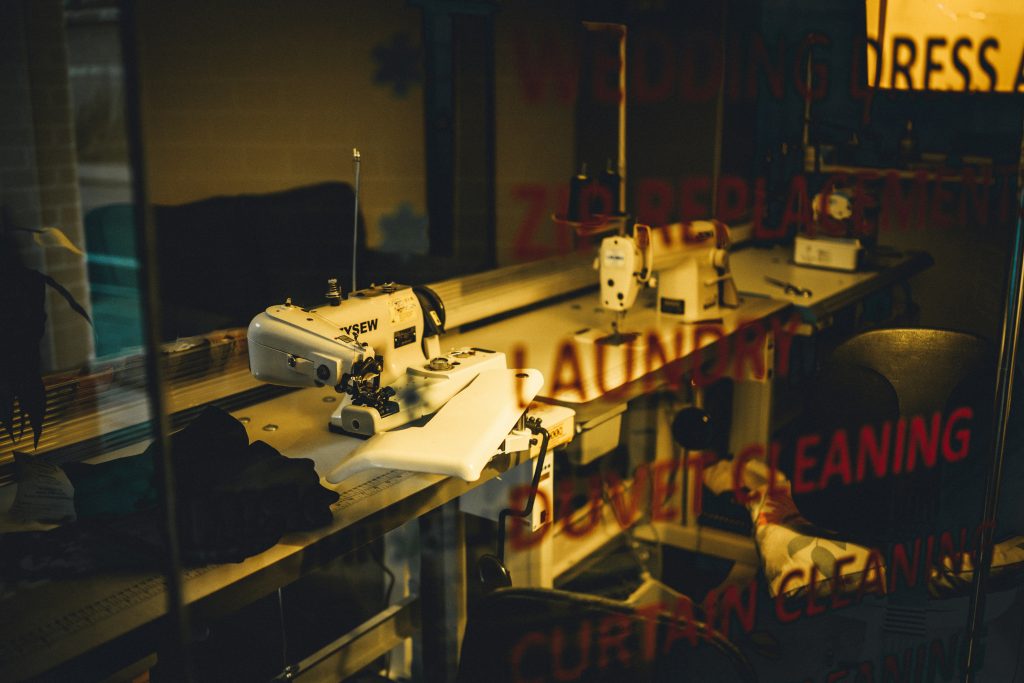
4 Real-World Examples of Biased AI
Facial Recognition:
1 Studies (e.g., by MIT’s Joy Buolamwini) showed that facial recognition systems misidentify darker-skinned and female faces at significantly higher rates.
Hiring Tools:
1 Amazon abandoned an AI recruiting tool that penalized resumes with the word “women’s” because it was trained on past hiring data favoring male applicants.
Credit Scoring and Lending:
1 Algorithms used by banks and fintech companies have been found to approve loans unequally by race or ZIP code.
5 Why Fairness in AI Is So Challengin
Fairness Is Contextual:
1 Different definitions of fairness exist: equal opportunity, demographic parity, individual fairness, etc.
Trade-offs Between Accuracy and Fairness:
1 Improving fairness may reduce predictive accuracy or increase false positives/negatives.
Opaqueness of AI Models:
1 Many AI systems, especially deep learning models, are “black boxes” that are hard to interpret or audit.
6 Can AI Be Made Fair?
Fairness-Aware Machine Learning:
1 Techniques such as bias mitigation during preprocessing, in-processing, and post-processing stages.
Diverse and Representative Data:
1 Better data collection practices to avoid historical imbalances.
Algorithm Auditing and Transparency:
1 Regular testing, impact assessments, and third-party audits.
Human Oversight:
1 Ensuring human involvement in high-stakes decisions to catch and correct AI errors.
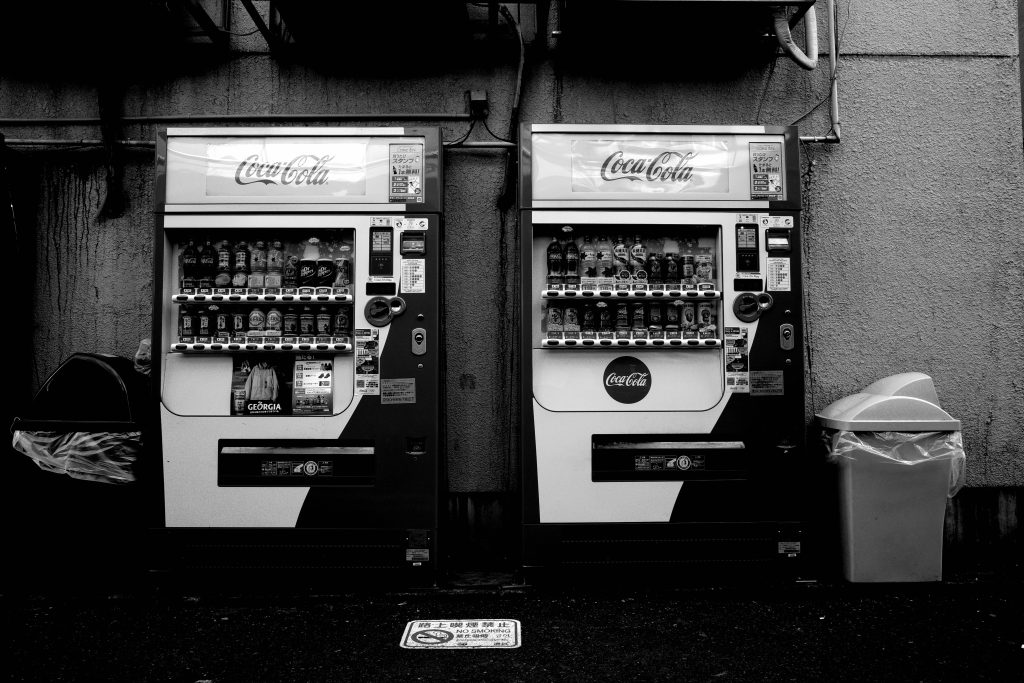
7 Ethical and Policy Considerations
Regulation:
1 Proposed laws (e.g., EU AI Act, NYC’s hiring AI law) demand transparency, explainability, and fairness.
Corporate Responsibility:
1 Companies must prioritize ethical AI development and adopt responsible AI frameworks.
Public Awareness:
1 Educating users and stakeholders about AI risks and how to question algorithmic decisions.
Conclusion
Final Thought: AI systems are only as fair as the humans who design and train them. Machines alone can’t be truly fair—but with intentional design, rigorous oversight, and ethical commitment, we can build AI systems that move us closer to equity rather than further from it.