Synthetic Data for AI Training
2 What is Synthetic Data?
Synthetic data is artificially generated data that mimics real-world data. It’s created using algorithms, simulations, or generative models (like GANs or diffusion models) instead of being collected from real-world events.
It can include images, videos, text, audio, tabular data, or even sensor readings.
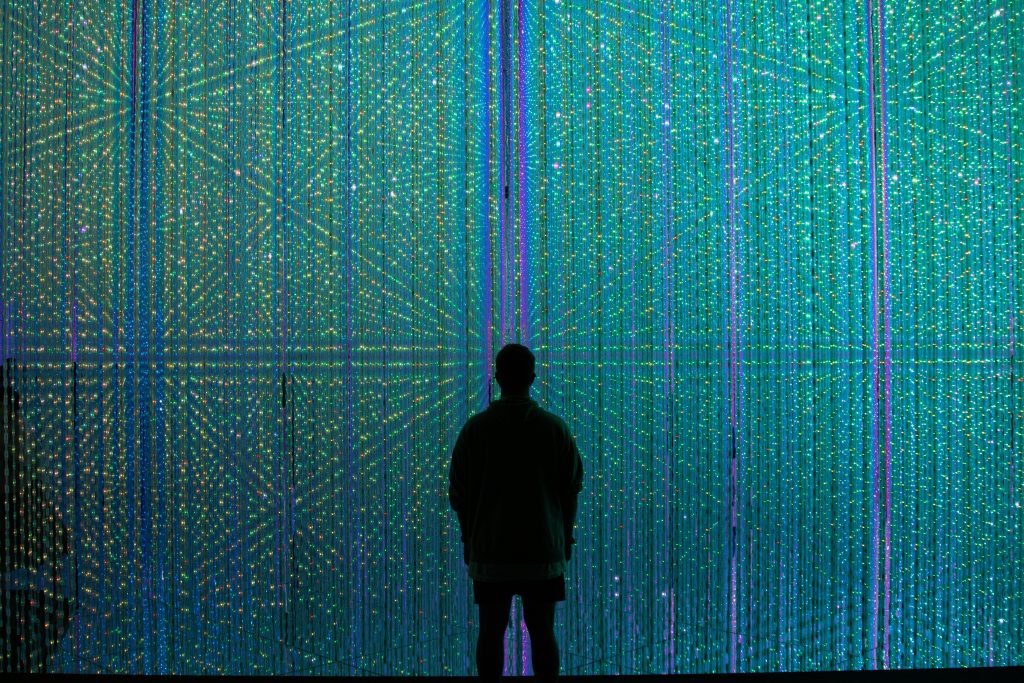
3 Key Use Cases
1 Computer Vision: Synthetic images for object detection, face recognition, medical imaging
2 Autonomous Vehicles: Simulated driving environments with rare obstacles or conditions
3 Healthcare: Synthetic patient records for research without violating privacy laws
4 NLP: Text generation for rare languages, domain-specific dialogs, or adversarial examples
5 Finance & Cybersecurity: Simulated fraud transactions or attack patterns
4 Challenges & Limitations
1 Realism: Low-quality or poorly designed synthetic data can mislead models
2 Overfitting to Synthetic Patterns: Models might learn artifacts specific to synthetic data
3 Validation Difficulty: Hard to benchmark accuracy without real-world ground truth
4 Regulatory Acceptance: Not all industries accept synthetic data as a valid substitute (yet)
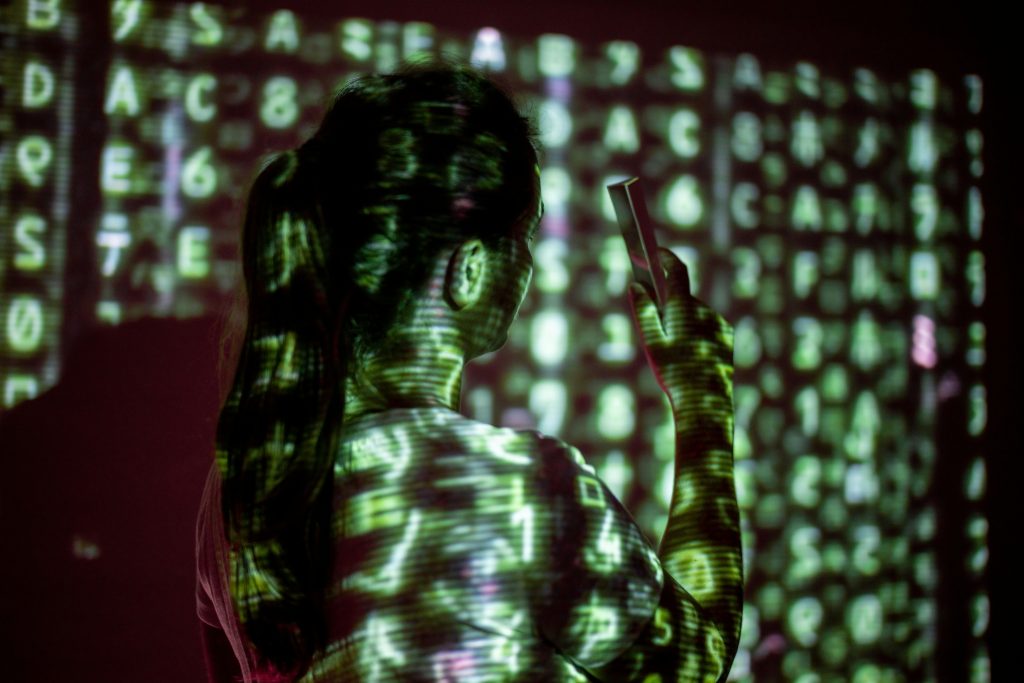
5 Future Outlook
1 Synthetic-to-Real (S2R) Transfer: Techniques like domain adaptation and domain randomization to bridge the gap.
2 Data-Centric AI: Focus on improving data quality (real or synthetic), not just model complexity.
3 AI-assisted Data Generation: Using LLMs and multimodal models to co-create synthetic datasets.
4 Standardization: Emerging frameworks and tools to validate and benchmark synthetic datasets.